Artificial neural networks based on metallic nanofibers
Project Director: Dr. Alexandru EVANGHELIDIS
Project code:
Project no.: PD 127
Acronym: NEURAWEB
Funding agency:The Executive Agency for Higher Education, Research, Development and Innovation Funding (UEFISCDI)
The purpose of this project was to create a small scale physical artificial neural network (PANN) by using electrospun metallic webs as a low-cost and easily fabricated substrate. This PANN could be used in portable applications for complex signal analysis, such as voice recognition, without requiring a constant Internet connection, thus enabling decentralized and private access to powerful machine learning services.
A reservoir computing network, also known as echo state network, is a class of artificial neural network algorithms which relies on a static randomly connected network, i.e. the reservoir, and a single trainable output layer, which greatly accelerates the training process, in contrast with the Deep Learning paradigm, where the network consists of multiple trainable layers. The particular morphology and nanoscale heat transport properties of electrospun metallic webs makes them well suited for use as reservoirs, as they offer both the required connectivity and the nonlinear response of the individual "neurons".
The activities of the project started at the micro level of individual fibers. Their electrical and heat transport properties have been measured experimentally and modeled using finite element methods. These results have then been fed into a macro scale model of the entire metallic web, which was used to find the physical parameters required for the PANN to work. Using those parameters, the PANN was fabricated and tested according to the standard literature benchmarks.
Contracted budget:
Principal Investigator: CS3 Dr. Alexandru Evanghelidis
Mentor: CS1 Dr. Lucică Miu
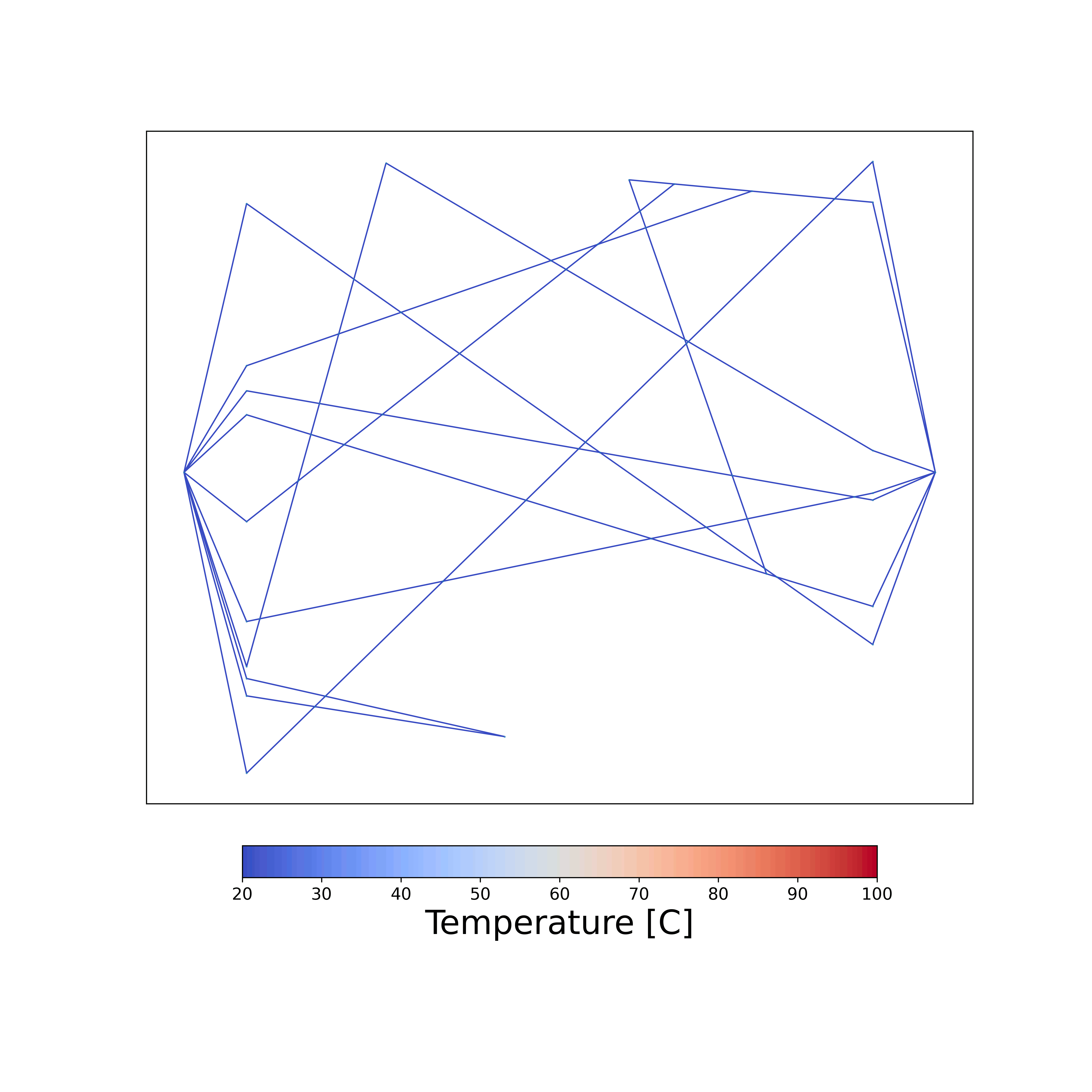
Example of a simulated sparse nanofiber network subjected to several voltage pulses which cause it to heat up unevenly.
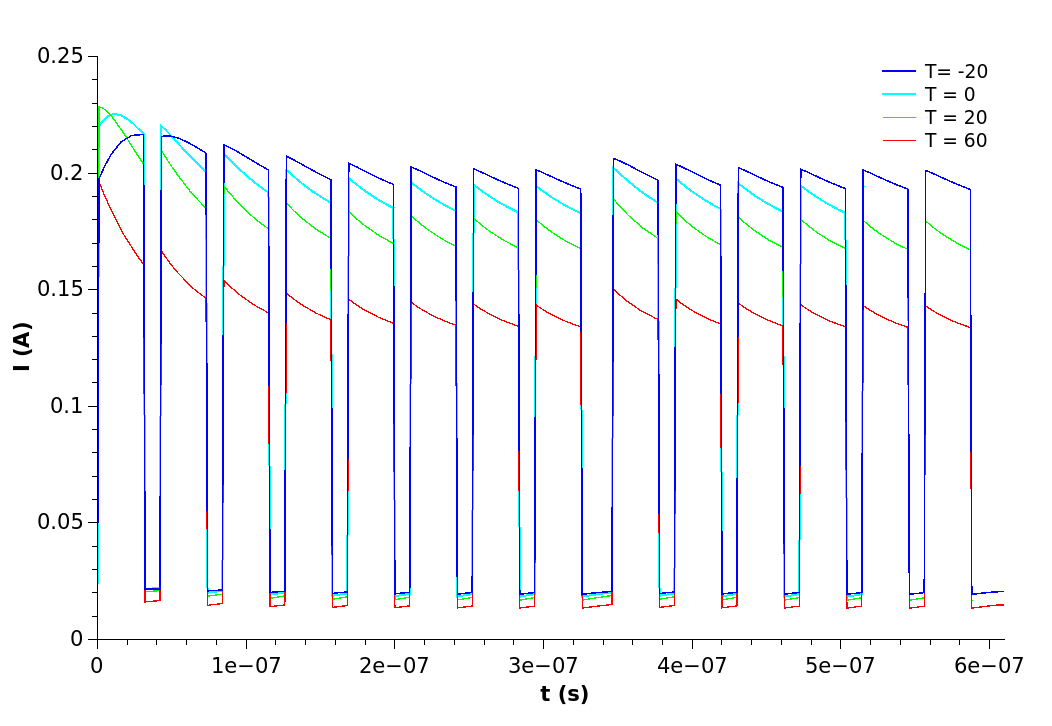
Influence of ambient temperature on the formation of current pulses in metallic nanofiber networks.
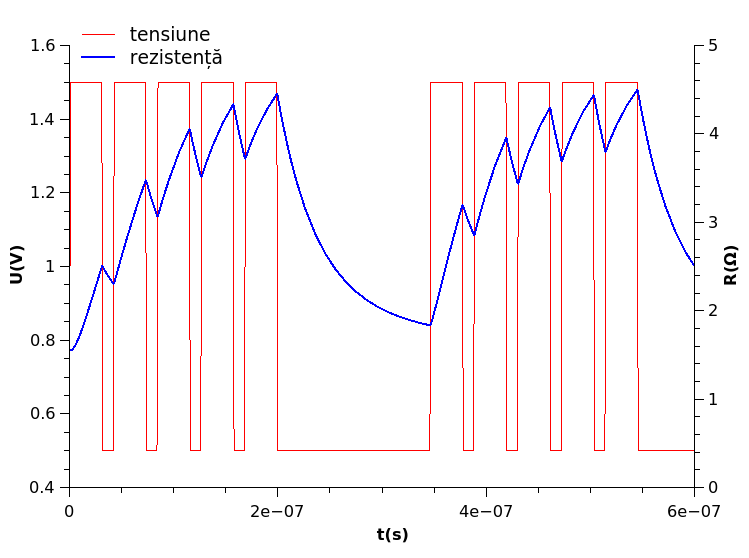
Leaky integrator behavior created in metallic nanofiber networks.
By defining an encoding protocol through which the target signal is transformed intro a train of heating micro-pulses, the heat capacity of the fibers becomes the computational equivalent of a short term memory, which gives the network the ability to “learn” from the stimuli to which it gets exposed to.
Electrospun fibers, covered with metal and transferred onto glass substrates.
Holder for the electrical contacting of the fiber network.
To obtain the results, an electronic interfacing device was designed, through which the metallic fiber network could be accessed and used in conjunction with machine learning algorithms, which were implemented on a regular desktop computer by using exclusively open source software (Python, NumPy, SciKit etc.). The interfacing device prototype was mad using a development board and other low cost, easily accesible, components. Despite its simplicity, the device was able to provide a good temporal resolution, as required by the experiments that needed to be run.
Schematic for the interfacing device.
By “tuning” the network with the right parameters, it was shown that it has the capacity to learn how to reproduce a complex signal.
Signal reproduced by the network after training on 100 samples of a target signal.
These results have proven that electrospun metallic nanofibers can be useful in machine learning applications, which opens some new avenues for further research and for the development of intelligent devices based on advanced materials. On the one hand, the full potential of this particular material has yet to be explored, as only the simplest possible configuration – one input and one output node, at macroscopic scale – has been tested so far. The microstructure of electrospun fibers, however, allows for a much larger number of contact points, which can considerably increase the amount of information that can be extracted from them, from which computational reservoirs can be created. On the other hand, both the knowledge and skills acquired during this project as well as, more pragmatically, the data acquisition & processing protocol that was developed, can be applied on other types of materials with known non-linear properties and behaviours.
PROJECTS/ NATIONAL PROJECTS
Copyright © 2025 National Institute of Materials Physics. All Rights Reserved